Are Tiger Cubs Evolving Into Tiger Quants?
Hedge funds influenced by Julian Robertson, including Coatue and Maverick, are exploring quantitative methods and systemic strategies.
A wise man adapts himself to circumstances, as water shapes itself to the vessel that contains it.
–Chinese Proverb
One family of fund managers dominates the hedge fund landscape like no other. Funds with ties to Julian Robertson’s Tiger Management, dubbed the Tiger Cubs, are some of the largest in the game and control $140B in aggregate market value, according to filings. But in the last few years, their investment style has faced pressure from a market environment that’s unfavorable to their fundamental strategies. In fact, an article in the WSJ labeled the Tiger family the “Prey of Wall Street,” implying that their style is out of favor, as quant strategies pick off much of the winnings that this market has to offer active managers.
After taking a closer look at the Tiger Cubs, we found that while many of them are experiencing a relatively tough period, the skills that have made them successful in the first place remain. When will the markets turn and see dispersion, volatility, and breadth come back (factors that impact fundamental equity strategies)? That’s anyone’s guess.
For now, some Tiger Cubs aren’t waiting around—they’re making changes to their process. A few (Coatue, Maverick, Viking) recently made headlines for implementing systematic strategies or employing quantitative methods to complement their traditional strategies. But after looking at the data, it seems only one manager from the lineage is actually taking the leap into quant strategies.
It’s important to make a distinction between tapping big data to improve an existing strategy and operating a systematic trading strategy.
The first strategy is really just adding more data points to enhance an existing processes, for instance: tapping into click data, satellite images of retailer parking lots, or credit card spending data to gain an edge on a specific trade. These alternative data sets help, but the trade is still made by a human considering the new input. It’s a relatively small step where a human is still the final decision maker (albeit with new data points on each potential investment). Interestingly, the result may be trading fewer securities. More data means more potential criteria to eliminate an investment from the long book and more time/resources to analyze existing investments.
The second approach uses a computer to find patterns in massive amounts of data indiscernible to humans, and trade on signals. The result is trading many more securities and holding them for shorter time periods. This is a huge departure for managers who, for decades, have relied on their intuition, experience, and judgement to manage capital.
Based on the information in the public domain, Viking and Coatue (and many others) seem to be taking the former approach while Maverick is taking the latter (with a portion of their capital).
The first approach requires a lot of data analysis; the PM’s trust in their recently hired data scientists, and perhaps some time to test the waters. According to Rado Lipuš, founder and CEO of alternative data scouting and research provider Neudata, managers face many challenges when integrating alternative data sets: “Researching and curating data providers requires time and resources as managers need to consider the quality, dependability, and accuracy of the data vendors.” And these days, there are many sources of alternative data. There’s also the problem of continuity. If a manager builds a process reliant on a data set that later becomes unavailable, they risk compromising the strategy. Still, Rado believes that most larger managers are either using or considering alternative data sets. Many of the smaller managers are still trying to figure it all out.
The second approach, operating a systematic strategy, requires something different. It requires relinquishing control. This may be why most managers in the Tiger lineage, with fundamental research in their DNA, are slow to dive in.
In today’s environment, the first approach would do little to adapt a strategy to the changes in the markets. For instance, low dispersion and high correlation within equities will still affect the potential rewards a manager reaps even if they get the trade right using all new data inputs. The second approach, I believe, could change the game entirely. Here’s why.
For years, upon analyzing managers’ trading behaviors, we’ve seen fundamentally driven managers lose money (and alpha) on their smallest positions. There are a variety of reasons for this, but here’s a simple illustration of our findings.
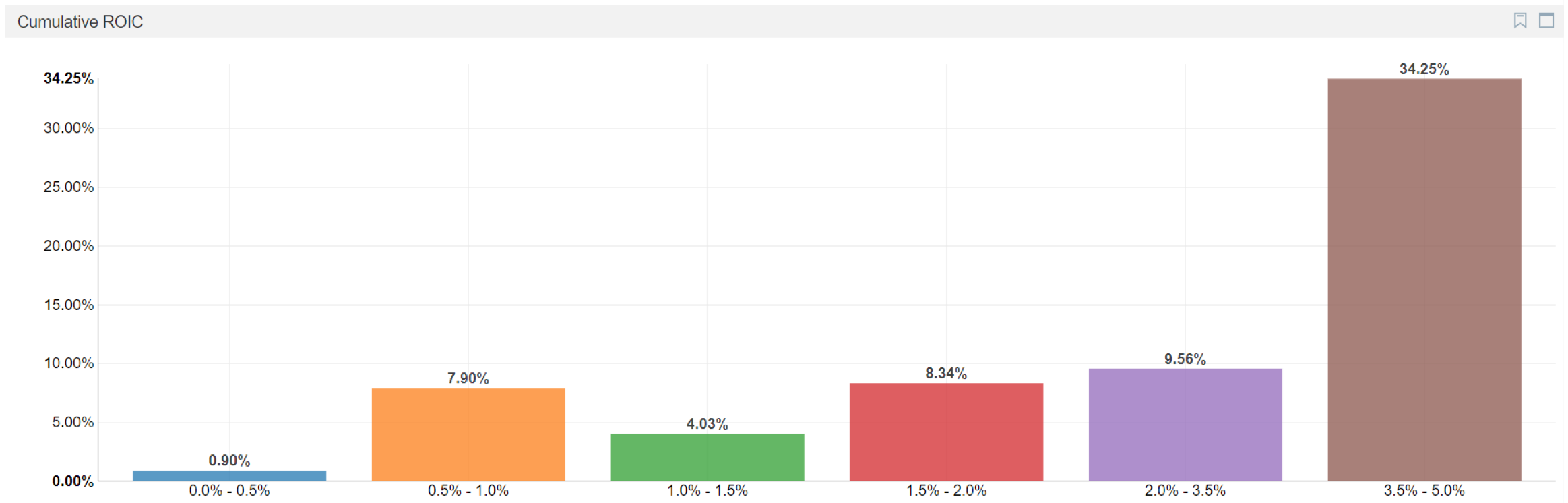
This is a typical profile of returns (ROIC) when broken out by position size buckets, smallest to largest. It plots the ROIC for buckets of the Novus Tiger Portfolio (all 50 managers, positions aggregated and weighted by reported value) over the last 24 months. Larger positions tend to have higher ROIC, and the smallest positions have the lowest ROIC.
This is true for other managers as well. Holding on to the smallest positions often translates into a drag on performance that, once quantified, warrants action.
So, if fundamentally driven managers figure out how to make money from their smallest bets, it could mean a significant return improvement. But there’s a caveat: this would only be true if the systematic strategy were part of the larger fund, or a complement to it. Run as a separate fund, it would only benefit the investors in that vehicle.
On the other hand, traditional quants only hold small positions. We rarely see a position of above 1% of holdings, as their trading strategies prefer a larger number of very liquid securities. They make money by trading around volatility on different signals, and quants rarely buy and hold for long periods.
Therefore, adding a quant layer on the smallest positions can be lucrative for fundamental mangers; they can turn a loss center into a profit center. (If they get the quant algorithms right, that is.)
Let’s compare the managers we mentioned to get a glimpse of their strategy when it comes to using data in some innovative ways.
Coatue vs. Maverick
Philippe LaFonte’s shop, Coatue, led a round of funding for Domino, an alternative data provider the fund employs, and has been hiring data scientists to take advantage of the new information. At the same time, Maverick’s Lee Ainslie has been raising money for internally run quant funds. Let’s look at the number of filed securities for both managers on their 13Fs, keeping our earlier statements about the effects of the two different approaches in mind:
Position Count: Coatue vs. Maverick
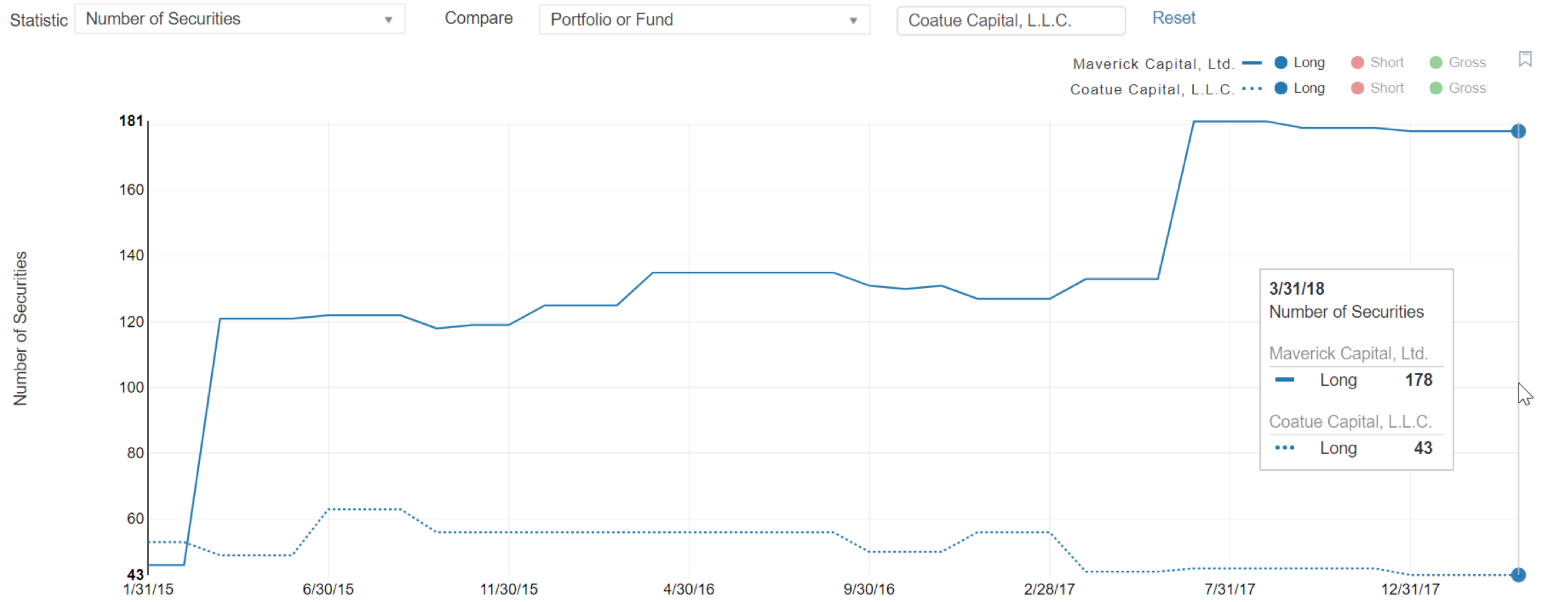
In 2015, Maverick filed less positions on their 13F than Coatue, and today they have 4X the number of names. You can also clearly see the “spikes” in position counts, presumably corresponding to the ramping up of their two quant funds in 2015 and last year. At the same time, the opposite is happening at Coatue; their position counts are decreasing.
Data also indicates that Maverick began to allocate meaningful capital to the quant strategies in January of this year. Maverick’s smallest positions now account for 15% of the publicly filed market value (sum of their total 13F positions),
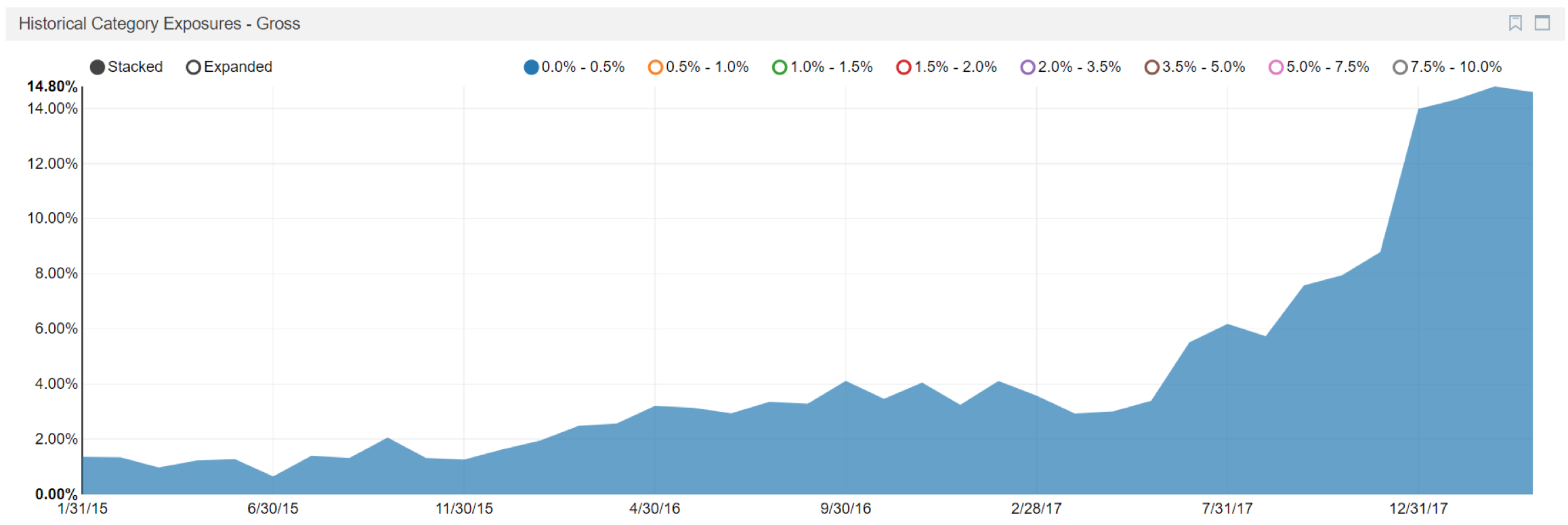
while Coatue’s smallest positions were slashed, as the fund shed lower conviction names:
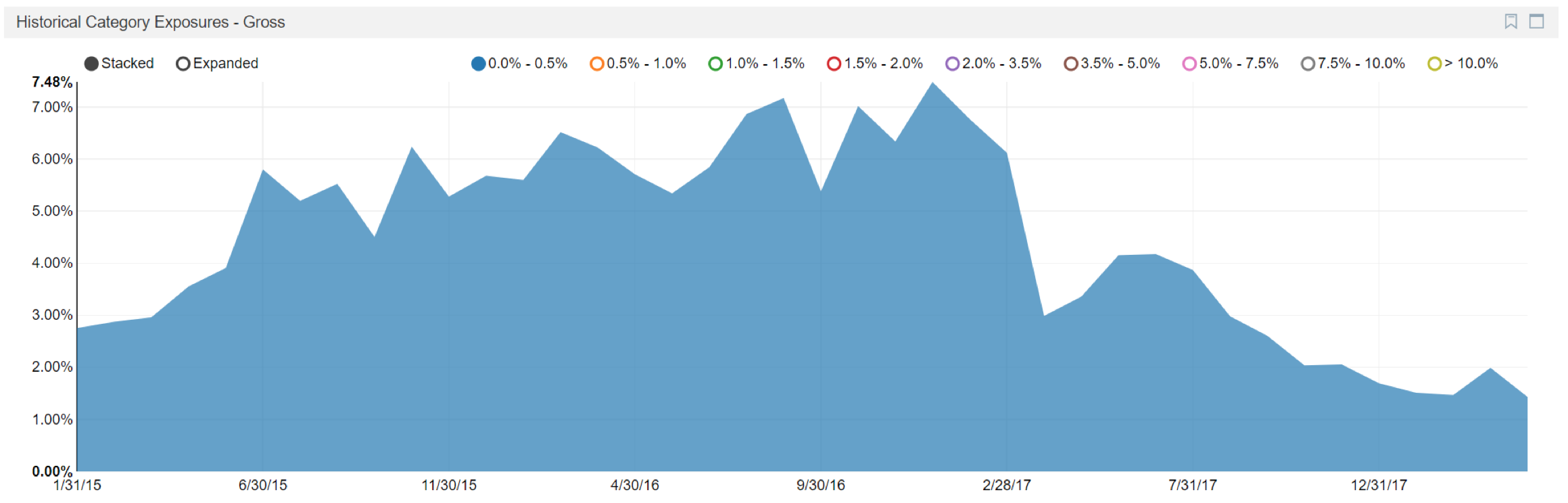
Even more evidence is seen by running overlap analysis on the two funds compared to a peer group of traditional quant managers.
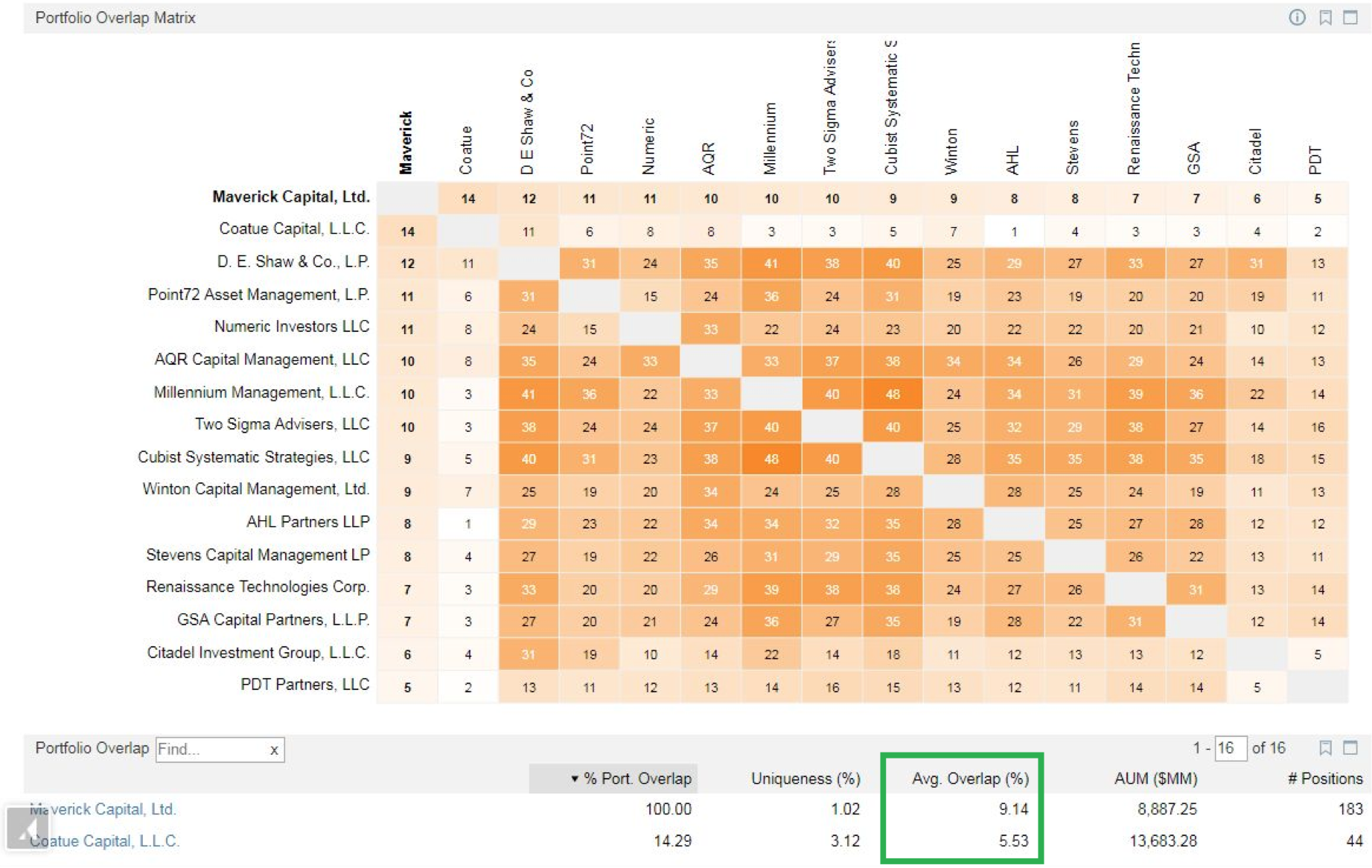
As we can see, Maverick’s portfolio overlaps more with the quants than does Coatue’s: 9.1% compared to 5.5% average overlap. The difference is relatively small, but what’s interesting is that this number increased for Maverick during the time they ramped up smaller positions. Just one year prior, both the managers’ numbers were at 5%.
While both managers are using data signals to enhance their returns, Maverick, true to its name, seems to be the only cub taking the plunge into the world of quant. Scrutinizing the position counts and concentration metrics for other Tiger Cubs, we see no evidence of quant strategies like Maverick’s in any of their peers. But it’s very likely that most cubs are doing something similar to Coatue with alternative data sets.
UPDATE June 11th 2018
At the date of the initial post, the news had not come out on Coatue’s systematic fund launch.
As of March 31st 2018 filings, it became evident that Coatue is experimenting with a different strategy – as their position count sharply increased.
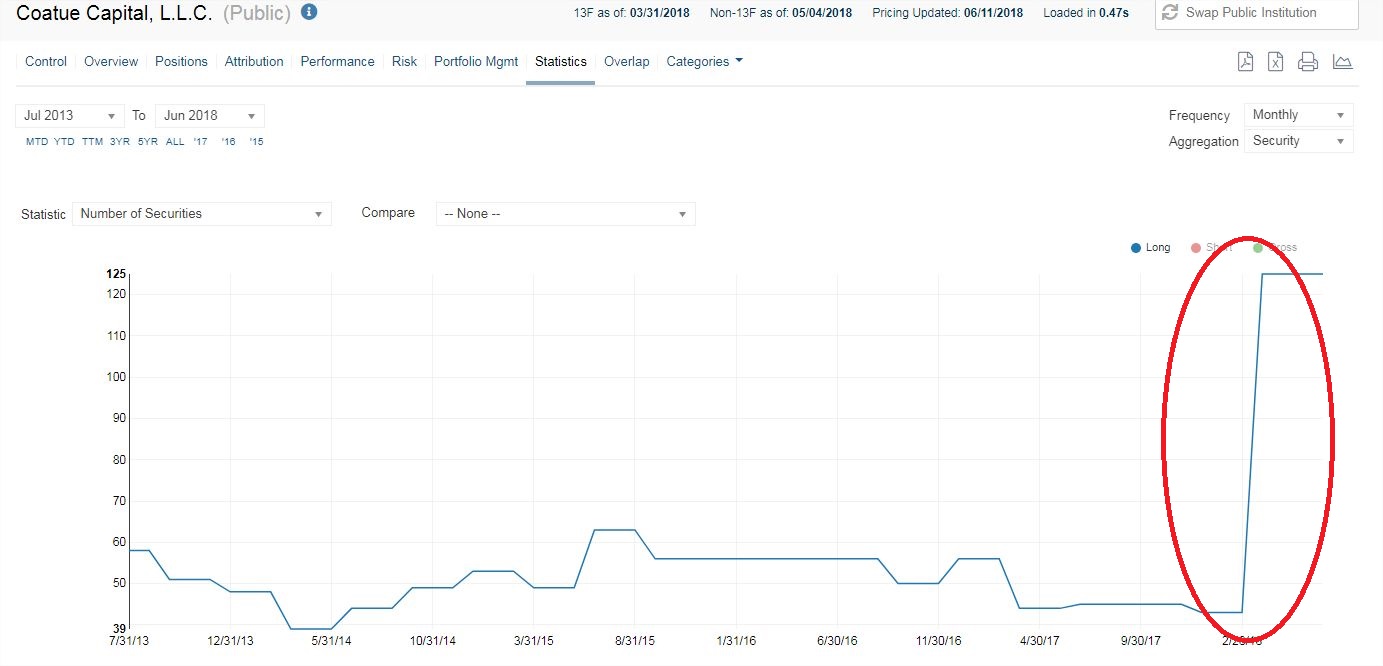
Still, data suggests that the manager has not deployed a lot of capital into the new strategy yet, as evidenced by their small capital allocation to their smallest positions. The overlap numbers have also not changed significantly.
We welcome any thoughts or questions on this data – please leave those in the comments below.